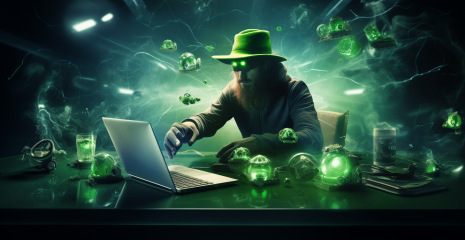
In the conditions of the rapid development of artificial intelligence technologies, criminals are increasingly resorting to the use of deep fakes – synthetic media created with the help of deep learning algorithms for fraud and extortion. These falsified videos and audios can be used to discredit public figures, manipulate public opinion and even extort funds. Detection of such attacks and protection against them requires special attention and the use of specialized cyber security techniques, which we will tell you about here
Deepfakes are synthetic media created using machine learning algorithms, named after the deep learning techniques used in the creation process and the fake events they depict.
Deepfake techniques cross disciplines and fields from computer science and programming to visual effects, computer animation, and even neuroscience. They can be convincingly realistic and difficult to detect if done well and with sophisticated and powerful technology.
But at the end of the day, machine learning is a fundamental concept for data scientists, and as such offers an interesting area of research in the context of deep fakes and the predictive models used to create them. The learning methods, algorithmic frameworks, and synthetic output of these models offer insight into deep learning and data.
Earlier in 2021, the FBI issued a warning about the growing threat of synthetic content, which includes deepfakes, describing it as “a wide range of created or manipulated digital content that includes images, video, audio, and text.” People can create the simplest kinds of synthetic content using software like Photoshop. Deepfake attackers are becoming increasingly sophisticated, using technologies such as artificial intelligence (AI) and machine learning (ML). Now they can create realistic images and videos.
Remember that cybercriminals do cyber theft to make money. Ransomware is usually successful. So it was a logical step for them to use deepfakes as a new ransomware tool. In the traditional method of ransomware distribution, attackers launch a phishing attack using malware embedded in an attractive deep fake video. There is also a new way to use deep fakes. Criminals can show people or companies all kinds of illegal (but fake) behavior that can damage their reputation if the images become public. Pay the ransom and the videos will remain private.
In addition to ransomware, synthetic content is used in other ways. Criminals can use data and images as weapons to spread lies and deceive or extort employees, customers and others.
Attackers can use all three of these attack styles together or separately. Remember, fraud has been around for a long time. Phishing attacks are already quite ruthless in their attempts to trick users. However, defenders are not paying enough attention to the rise of AI/ML to spread disinformation and extortion tactics. Today, criminals can even use programs designed to create pornographic images from real photos and videos.
Users have already become victims of phishing attacks, so detecting deep phishing attempts has become even more difficult for ordinary users. It is important that security programs include cybersecurity training as a mandatory element. This training should provide information on how to distinguish fake messages from real ones.
This task may not be as difficult as it may seem. Phishing attack technology may be quite advanced, but it is not perfect. In one of the webinars, Raymond Lee, CEO of FakeNet.AI, and Etai Maor, Senior Director of Security Strategy at Cato Networks, explained that one of the key features for detecting fakes is the face, and especially the eyes. If the eyes look unnatural or the facial features don’t seem to move, it’s probably an altered image.
Another way to distinguish deepfakes from real information is to apply best practices in cybersecurity and adopt a philosophy of zero trust. It’s important to check all the data you receive. Double and even triple check the source of the message. If necessary, use image search to find the original if possible.
When it comes to your own images, use a digital signature or watermark to make them harder to forge.
In general, existing security systems can be applied to prevent phishing and social engineering attacks. Deepfakes are still in their early stages as an attack method, so cybersecurity teams have an advantage in preparing defenses as the tools to detect and defend against these attacks improve. It is important not to allow these threats to minimize our peace of mind.
For academics and data professionals interested in the impact of deepfake technology on private enterprise, government agencies, cybersecurity, and public safety, studying the methods of creating and detecting deepfakes can be extremely useful. Understanding these methods and the science behind them makes it easier to respond to the potential threats associated with the harmful use of synthetic media.
With the development of deep learning models, it becomes important to develop the skills and resources to detect and prevent the potential threat that comes from the malicious use of deepfakes. This can be an important task for researchers, companies and the public.
Government institutions and large corporations allocate significant funds to the development and improvement of deepfake detection systems. Such investments can help reduce the risks associated with the large-scale spread of false information and disinformation. The models being created by researchers like Thanh Thi Nguyen and his colleagues could be important tools for detecting and combating deepfakes in the future.
The first-order motion model is an interesting and advanced approach to image animation. This model is trained to reproduce motion based on input data and create animations that allow users to animate videos or create new scenes based on existing data.
The authors of the model taught her to “reconstruct” educational videos by combining one frame and the studied representation of movement in the video. This allows the model to understand how objects move in the video and use this information to create new frames or animations.
Dimitris Poulopoulos, a machine learning engineer, used this model to create interactive scripts and animations. He shared source code and use cases, allowing other users to experiment with the technology.
The application of this model can be diverse, from the creation of visual effects in movies and video games to the animation of media content. It is an essential tool for display creators and video editors looking for new ways to create engaging content.
Viewing the tooltips in a piece of media is a starting point, but it’s not enough. We also recommend running a side search to confirm or deny the accuracy of the video, something you can also do at home. According to a fact-checking guide by Mike Caulfield, a research fellow at the University of Washington’s Center for an Informed Public, lateral searching means reading “many related sites instead of digging deep into a specific site.” Open multiple tabs in your web browser to learn more about the claim, who is spreading it, and what other sources are saying about it.
Caulfield advises, “Go off the page and see what other authoritative sources have said about the site,” and pull together “different pieces of information from the Internet to get a better picture of the site.”
If Biden’s audio recording of the bank failure was real, the news would almost certainly have covered it. But when we searched, the results only included other social media posts sharing the clip or news articles debunking it. Nothing has confirmed that this is true.
Similarly, when PolitiFact found a video of DeSantis announcing his 2024 presidential run, no reliable news source confirmed it — something that would have happened if DeSantis had actually announced.
“It’s important to note, first of all, whoever is sharing this video, you know, look for a little bit of the origin of where this video was originally from,” Liu said. “If the message really matters to the audience, they should look for cross-validations.”
Fact checkers also use reverse image searches, which social media users can also do. Take screenshots of videos and upload them to sites like Google Images or TinEye. The results can reveal the original source of the video, whether it has been published in the past, and whether it has been edited.